Speech Synthesis
Exploring Speech Synthesis in CALL: Enhancing Language Learning through Technology
Speech synthesis, also known as text-to-speech (TTS), is the artificial production of human speech. It converts written text into spoken words using computational processes, generating audio that closely mimics natural speech patterns. Over the years, this technology has evolved from producing robotic, monotonous voices to generating more natural and expressive speech, thanks to advancements in deep learning and linguistic modeling. This progress has significantly expanded the role of TTS in Computer-Assisted Language Learning (CALL), where it enhances listening comprehension, pronunciation practice, and overall engagement with spoken language. The TTS can also generate and augmented training data for its downstream tasks – Automated Speech Recognition.
Speech Synthesis Applications in CALL
The integration of speech synthesis into CALL platforms has revolutionized language learning by offering dynamic and interactive tools. It has been commonly proven the effectiveness of speech synthesizer on L2 acquisition of writing (Kirstein, 2006), vocabulary and reading (Proctor, Dalton, & Grisham, 2007) and pronunciation (Cardoso, Collins, & White, 2012; Soler-Urzua, 2011). Building upon this research, a systematic review by Widyana et al. (2022) further highlights that Text-to-Speech (TTS) technology holds significant potential for enhancing language learning by improving language skills, supporting students with disabilities, and increasing motivation, while also facing limitations such as lack of naturalness, emotional expression, and real-time interaction.
A growing research focus is on personalizing and characterizing speech synthesis technology for improved language learning outcomes. One notable example is Duolingo, a widely-used language learning application that employs TTS to give voice to its characters, enhancing user engagement and providing learners with accurate pronunciation models. This approach allows learners to hear and practice new vocabulary in context, facilitating better retention and understanding.
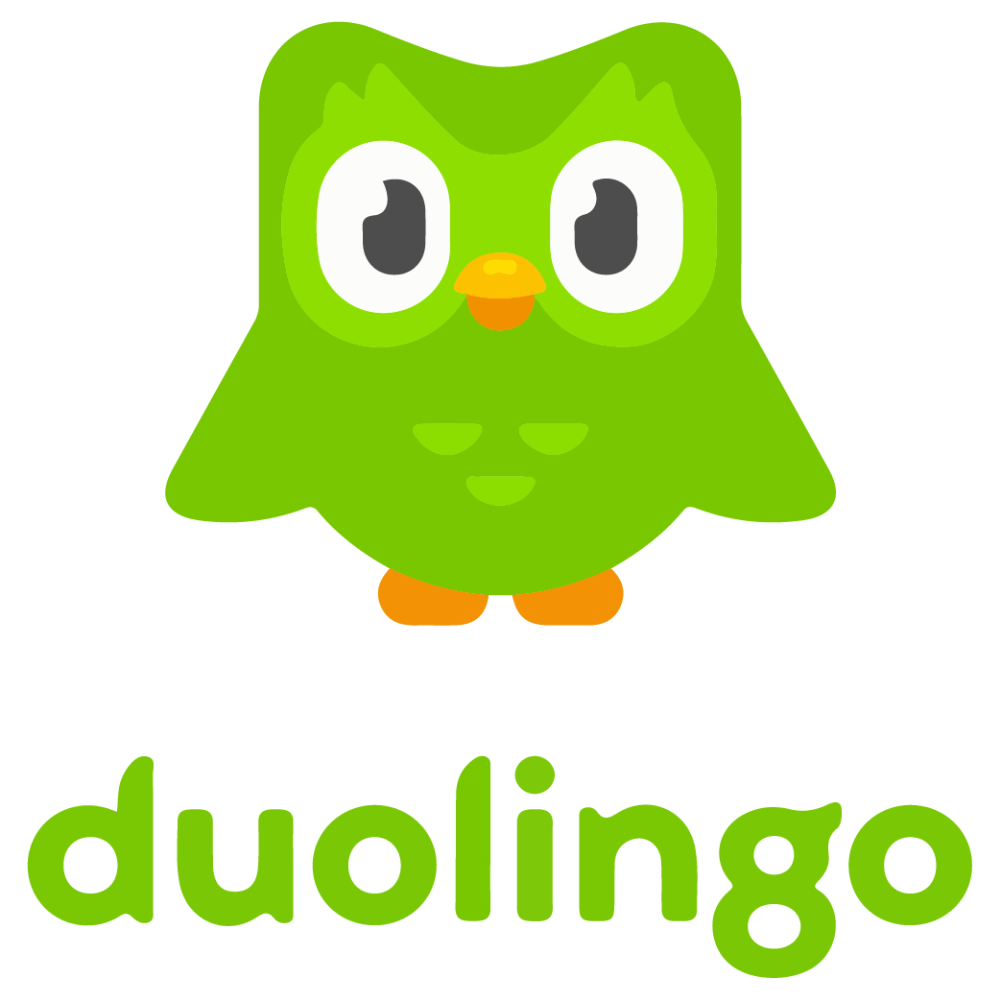
Watch this Video
Another innovative application is the Learning And Reading Assistant (LARA), which utilizes TTS to aid in reading comprehension. LARA transforms written text into spoken words, enabling learners to simultaneously see and hear the language. This multimodal input supports the development of listening skills and reinforces the connection between written and spoken language forms.
Empirical studies have highlighted the pedagogical benefits of TTS in pronunciation training. Research indicates that TTS provides accessible and personalized input, allowing learners to engage in effective practice outside traditional classroom settings. For instance, studies have demonstrated that TTS can facilitate the acquisition of second language (L2) pronunciation through shadowing and listen-and-repeat exercises, leading to improved speech production.
Modern TTS engines, such as Amazon Alexa, Google Assistant, and Apple Siri, can even incorporate emotional tones like enthusiasm or disappointment, making them more engaging for educational purposes. Studies suggest that using enthusiastic TTS voices can positively influence learners' emotions and cognitive load in multimedia learning environments.
Co-Joint Use of Speech Synthesis and ASR
The combined use of speech synthesis and ASR technologies offers a comprehensive approach to language learning. ASR systems can transcribe spoken language into text, enabling learners to receive immediate feedback on their pronunciation accuracy. When integrated with TTS, learners can engage in interactive dialogues, where they listen to synthesized speech and respond verbally, creating a simulated conversational environment.
One specialized branch of CALL is Computer-Assisted Pronunciation Training (CAPT). CAPT systems utilize TTS to provide pronunciation models and ASR to assess learner speech, identifying errors and offering corrective feedback, ideally. However, the scarcity of speech data poses a significant challenge for model training, particularly in detecting pronunciation errors or supporting low-resource languages. To address this problem, researchers have proposed generating synthetic mispronunciations through TTS, augmenting the training data and enhancing the system's ability to detect and correct pronunciation errors.
Conclusion
This blog has primarily explored the role of speech synthesis (TTS) in Computer-Assisted Language Learning (CALL), highlighting its applications in pronunciation training, reading comprehension, and interactive learning. As speech synthesis technology continues to evolve, its potential in language education will only grow, making learning more accessible, efficient, and immersive.
Click here to see more functions of speech synthesis in our life: "How Voice Synthesis Can Improve User Engagement in Apps"
Earlier references:
1. Kirstein, M. (2006). Universalizing universal design: applying text-to-speech technology to English language learners’ process writing. Doctoral dissertation. University of Massachusetts, U.S.A.
2. Proctor, C. P., Dalton, B., & Grisham, D. L. (2007). Scaffolding English language learners and struggling readers in a universal literacy environment with embedded strategy instruction and vocabulary support. Journal of Literacy Research, 39(1), 71-9.
3. Cardoso, W., Collins, L., & White, J. (2012). Phonological input enhancement via text-to-speech synthesizers: the L2 acquisition of English simple past allomorphy. Paper presented at the American Association of Applied Linguistics conference, Boston, U.S.A.
4. Soler-Urzua, F. (2011). The acquisition of English /ɪ/ by Spanish speakers via text-to-speech synthesizers: a quasi-experimental study. Master's Thesis. Concordia University, Montreal, Canada.